The amusement as well as media (E&M) business is actually a diverse sector composed of several segments such as media, television, and film streamed online. By 2021, the U.S. E&M business is projected to reach $759 billion in revenue, increasing at a compound annual growth rate (CAGR) of 3.6 percent.
Despite the anticipated growth, there are concerns about a revenue declines in more traditional market segments. Being a result, business analysts like PwC argue that user experience need to take going up priority and AI is actually among top emerging technologies poised to positively add to our energy.
To evaluate the emerging job of AI in the E&M business, we explored this industry in depth to help you answer questions industry leaders are actually asking these days, including:
- What kinds of AI apps are currently being used in the entertainment as well as media business?
- Just how has the industry responded to these AI uses?
- Will there be any typical trends with these innovation efforts – and just how could these trends perhaps impact the future of the entertainment as well as media sector?
Within this document we break down uses of artificial intelligence of the entertainment as well as media business market to offer company leaders with an understanding of present and emerging trends that could influence the sector of theirs. We will start with a synopsis of the sectors we covered:
Entertainment and Media AI Applications Overview
According to the assessment of ours of the uses in that industry, the vast majority of entertainment as well as media use cases seem to fall into 3 major categories:
- Marketing and Advertising: Companies are training machine learning algorithms to help develop film trailers and design advertisements.
- Personalization of User Experience: Entertainment providers are using machine learning to recommend personalized content based on data from user activity and behavior.
- Search Optimization: Media content producers are using AI software to improve the speed and efficiency of the media production process and the ability to organize visual assets.
In the full article below, we’ll explore the AI applications of each application by section and provide representative examples.
Marketing and Advertising
Fox and IBM Watson – Morgan Film Trailer
In August 2016, IBM announced the release of the trailer for a 20th Century Fox suspense/horror film Morgan reportedly produced using machine learning. The study team trained the AI program on scenes from “100 horror movies.” Features from every one of the film scenes have been categorized into what the staff known as “moments” and were then analyzed based on visual, audio & scene composition components.
After the method received an understanding of the kinds of scenes present in a regular suspense/horror film trailer, it was provided the full length movie and then recommended ten moments for the Morgan trailer. A total of 6 minutes of footage was pulled out of the 90 minute video, leading to a 24 hour process, from start to finish. Comparatively, the film trailer growth process usually takes many days to complete. See the entire movie trailer below:
Even though the effect of integrating AI might have resulted in savings in trailer production costs, the estimated eight dolars million budget film grossed just more than $7.3 million in worldwide box office sales. Nevertheless, with Morgan being the very first attempt at using AI for trailer growth, it’s too early to effectively determine the immediate effect on ticket sales.
This particular software is actually akin to IBM Watson’s foray into sports – the place that the engineering was utilized to make a highlight reel from tennis matches by analyzing video footage as well as fan reactions in time that is real out of several angles (we covered this alternative Watson use case in our full AI in Sports article).
McCann Erickson Japan – “AI Creative Director”
In March 2016, advertising agency MaCann Erickson Japan reportedly launched an AI imaginative director referred to as AI CD ß. The company claims this’s the very first robotic innovative director created using artificial intelligence. AI-CD ß was officially hired on April one, 2016 along with eleven other man staff.
The machine learning algorithm steering the AI worker was taught on information including certain components of TV shows as well as about a decade’s worth of precise info on the winners of All Japan Radio & Television Commersion Confederation’s CM Festival. Through data mining, the device is able to draw out ideas as well as themes which would match a specific client’s ad campaign.
In September 2016, AI-CD ß was pitted against McCann Erickson Japan imaginative director Mitsuru Kuramoto, in a pleasant duel. Tasked with creating a spot for a Japanese mint brant, advertising “instant, durable refreshment that lasts for ten minutes,” each entry was submitted for judging by a nationwide poll.
The poll results reportedly showed that Kuramoto received the vast majority of the vote coming in at fifty four % while AI CD ß earned the remaining forty six %. Although this is not the perfect effect for the AI creative director, the narrow margin shows promise for the robot’s succeeding work.
Nevertheless, it’s essential to accept the inherent limits of AI in producing first concepts with no man assistance. Tony McCaffey, PhD a cognitive psychologist as well as computer scientist by instruction, conducted research reportedly demonstrating mathematical evidence that computer systems are actually restricted in the power of theirs to do inventive activities. He’s among analysts that argue that while repeated chores could be better handled using AI, with regards to imagination, man and computer collaboration is best.
Personalization of User Experience
Netflix – Machine Learning Workflow Management
With regards to on demand entertainment, personalization of the user experience has shifted out of a luxury to a user expectation. For instance, based on its 2016 yearly article Netflix boasts 93 zillion worldwide members streaming more than 125 million hours of Tv programs as well as films a day . Predicting what a user would like to watch is actually a vital component of the company’s business model. Machine learning is reportedly fundamental to streamlining the range of user preferences.
In May 2016, Netflix announced the development of a workflow management and scheduling software called Meson to reportedly deal with its different machine mastering pipelines which “build, train, and validate personalization algorithms” responsible for supplying video suggestions.
For a deeper look into recommendation systems, readers may find Use Cases of Recommendation Systems in Business – Current Applications and Methods to be a useful resource.
IRIS.TV – Recommendation and Personalization
Reportedly driven by printer learning, IRIS.TV offers a B2B service to help businesses in tracking & improving client interaction with the digital content of theirs. Examples of media business consumers include Hearst Digital Media, CBS and also the Hollywood Reporter.
In the four: 25 minute job interview below, IRIS.TV founder Field Garthwaite covers the platform as well as his company’s cooperation with IBM Watson, which can help to operate the machine learning capabilities:
Specifically, the platform is designed to complement content to users based on the preferences of theirs. The application combines into the vast majority of video players. The algorithms “learn” what users wish to look at as well as suggests related content.
In one case study, the company reports that The Hollywood Reporter Billboard Media Group created a fifty % increase in viewer retention with a period of 3 months.
Search Optimization
Zorroa – Machine Learning for Visual Asset Management
Zorroa offers a platform for handling visual assets which reportedly combines machine learning algorithms to enable users to do content searches inside big databases. Documents are actually imported into what’s known as an “analysis pipeline.” The pipeline is actually made up of processors which tag each visual advantage. Algorithms are taught to identify certain ingredients of visual content which could likewise be structured as well as catalogued to provide strong search engine results. Examples include:
- Face Recognition (find faces and determine whether they’re known, if so tag the advantage with a name)
- Image Classification (use a neural network to classify the image into a set of predefined categories)
After the analysis pipeline is actually operating, the process remains with workflow management measures allowing users to scale up the task for big datasets and fine tune categorization of visual assets. The picture below depicts a visual representation of the process:
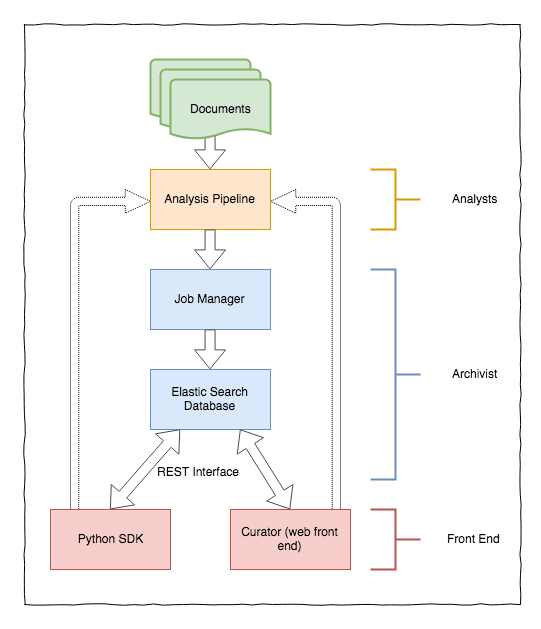
In April 2017, Zorroa Corporation announced the launch of its machine learning platform which would reportedly allow people to do searches and run analytics on visual assets located within big databases. Zorroa calls the device the Enterprise Visual Intelligence (EVI) platform.
The company claims that Sony Pictures Imageworks applications Zorroa EVI to “analyze as well as monetize millions of visual assets” which have been created through the years. For instance, in one case learn, Sony Pictures claims that a certain video search which would usually take twenty seven hours was finished in only three minutes with the EVI platform.
The short 58 second video below demonstrates how the video search was performed in the EVI platform:
Inside another example, the company reports it assisted a client in the oil as well as gas business tag and organize PDFs and photos to finish a mergers as well as acquisitions (M&A) valuation. Zorroa’s statements it reduced the processing time from three weeks to one month and enhanced the discoverable visual assets from ten % to ninety %.
Presently, it seems that the business is targeting clientele in the E&M as well as gas and oil industry. A platform of this particular type would formally be helpful for any organization where accessing and planning big databases of visual information is a typical job.
Concluding Thoughts
Emerging uses of machine learning in the entertainment as well as media business have been put on to several parts of value for businesses such as marketing, advertising as well as content creation. Innovations poised to provide the best advantage over time is going to save business professionals time and boost efficiency of the production process.
We are going to provide the ideas of ours on which uses in the following paragraphs seem to hold the most promise based on our research. mostly ranking each from most practical to least viable:
Personalization of the User Experience
Leaders and emerging competitors in the on demand entertainment room are actually leveraging machine learning to maintain the power of theirs to personalize content at scale for each customer. Given that personalization is actually turning into a regular client expectation, AI is actually poised to become an important approach for keeping pace with customer need.
Businesses centered on personalization of the user experience are actually showing up to deliver value for the clientele of theirs in the on demand entertainment room. As contests increases of that industry, evidenced by YouTube’s advertising push of YouTube Red, machine learning will be increasingly vital.
Search Optimization
Zorroa Corporation’s Enterprise Visual Intelligence (EVI) platform is actually fulfilling an useful necessity for film production houses, helping to enhance the procedure of looking for certain visual assets. In case the EVI platform regularly provides the outcomes described by Sony Pictures, it’s the potential to be generally used to the film business.
Marketing and Advertising
In comparison, the present lack of details on a correlation between machine learning in film trailer developement and ticket sales causes it to be difficult to anticipate how fast other films might follow suit. Nevertheless, if the hassle for the Morgan film was meant much more as a marketing strategy, media buzz was in fact produced.
Nevertheless, the movie business wants equipment which reduce generation time as well as costs. Consequently, we are able to count on feedback in the coming years on which of those apps might prove very helpful. These information might substantiate the great AI can provide to the market in several capacities of the production process.
The challenge of applying AI for much more innovative jobs, as in the situation of McCann Erickson Japan’s AI creative director, might require a lot more analysis before competing firms make investments in this particular technology. For maximum results, seasoned resourceful directors are going to need to get constantly interested in the AI instruction as well as advancement method which might cost you advertising and marketing agencies worker time.
Source: This is originally published by Kumba Sennaar ,emarj.com